Although they are relatively new technologies, machine learning (ML) and artificial intelligence (AI) are by no means novel. In the past, machines have performed a variety of chores and duties that people were thought to be too trivial and unclean to perform. These robots have developed over time to perform more sophisticated and difficult tasks, such as formulating strategies and making decisions. The need for people to have faith in these robots’ strength rather than cast doubt on it, however, is growing as complexity rises.
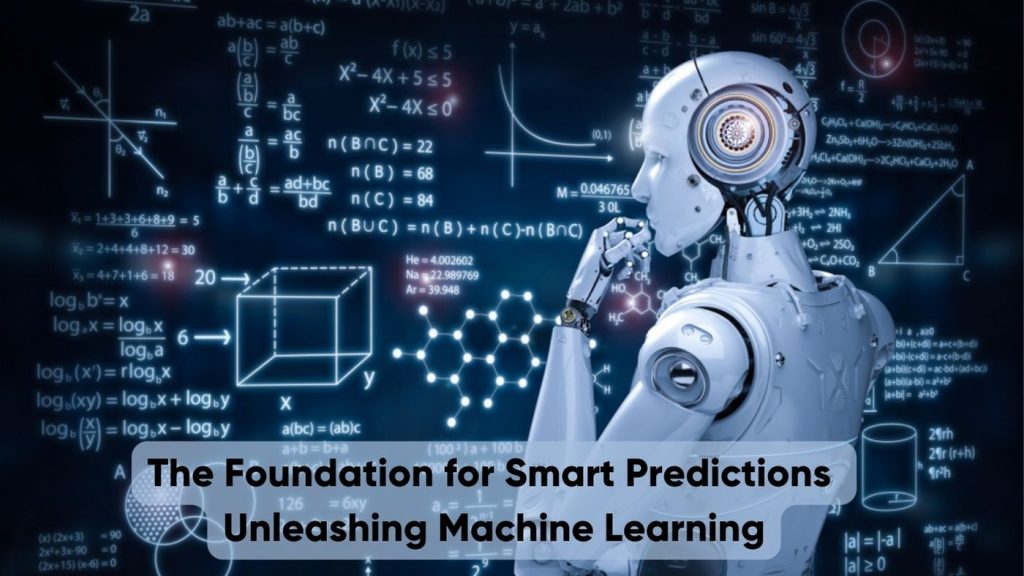
According to a recent study, machine learning, particularly deep learning, is rapidly gaining ground in workplaces across industries. For instance, hundreds of businesses in the healthcare sector use machine learning algorithms and predictive analytics to speed up the discovery of new drugs and identify illnesses from medical pictures. Similar to how self-driving cars utilizing ML are likely to become commonplace in the transportation industry within the next few years, with commercial uses of these cars following closely behind.
How does machine learning work?
In order to help businesses process massive amounts of data for producing precise suggestions, these intelligent systems take on low-level pattern identification tasks including picture recognition, speech recognition, and natural language processing. ML enables computer programs to make precise predictions with little room for error.
But because the core algorithms used in this decision-making process are frequently hidden from company employees, ML systems function as shunned “black boxes.” The increased likelihood of bad decisions being made by machines and the associated costs discourages enterprises from giving them fundamental competencies.
According to research, robots will have to give more detailed justifications for their recommendations in the years to come. This contact will allow individuals to comprehend and behave responsibly as the subsequent step of human enhancement by machines. It will work to put humans and machines on an efficient team.
Synergy in Machine Learning
ML-powered intelligent systems are now available to collaborate with human coworkers. Organizations can promote collaboration and efficiency in the workplace by implementing smart devices for accountability, fairness, and transparency. However, these futuristic highly intelligent devices won’t take the role of individuals. In a way that enables businesses to make decisions that are wiser, better, and more accurate, they will help and complement humans.
For advanced ML-led systems, there are three primary market factors. First, it is crucial for businesses to be transparent about how personal data is used for hiring and other decision-making, as mandated by legislation like the EU’s GDPR. Second, systems must be able to clearly communicate the reasoning behind their decisions in order to gain the trust of humans and AI. Third, there’s a need for improved machine-human interaction. The businesses of tomorrow will increasingly require both resources to work together, as algorithms get better at seeing little patterns in massive amounts of data and people get better at tying together high-level patterns.
So how exactly might machine learning improve the way that outputs are explained? Three different approaches have been found in the research to do this:
Data-level explanation – By making comparisons with other cases, ML-based systems can use this technique to demonstrate the modeling and its outcomes. This enables the system to defend any choice made on a specific problem or targeted forecast.
Model-level explanation: In this method, machine learning algorithms are the main focus. By putting a layer of subject-matter expertise on top, this approach makes the argument more intelligible to people. Model-level explanation, when compared to other approaches, abstracts the most from the data through rules or by fusing it with semantics.
Hybrid-level explanation: This method is most effective and helpful when the data being studied is especially big, intricate, or dense. By restructuring data at the level of the metadata, the approach employs a high level of abstraction. As opposed to other methods, which rely on the data as evidence, the hybrid-level explanation provides a metadata-level explanation for each feature.
ML will be improved to enable complex systems to:
- Summarize the thinking underlying their findings and how they came to them.
- Outline the system’s advantages and disadvantages.
- Compare their production & performance to other intelligent devices.
- convey outcomes in a thorough way that illustrates the possibilities of the next technology.
- Make company decision-making more intelligent.
The significance of machine learning
Even though there are constantly new technologies emerging, machine learning is still utilized in a number of different industries.
Machine learning is significant because it aids in the development of new goods and provides businesses with a picture of trends in consumer behavior and operational business patterns. A significant portion of the operations of many of today’s top businesses, like Facebook, Google, and Uber, revolve around machine learning. For many businesses, machine learning has emerged as a key competitive differentiation.
Machine learning has a number of real-world uses that produce tangible business outcomes, including time and money savings, that could have a significant impact on your company’s future. Particularly, we observe a significant impact on the customer care sector, where machine learning is enabling humans to complete tasks more quickly and effectively. Machine learning automates actions that would usually require a human person to complete them, including resetting a password or checking an account’s balance, through Virtual Assistant solutions. By doing this, valuable agent time is freed up so they can concentrate on the high-touch, complex decision-making tasks that humans excel at but that machines struggle with.
By using our own Adaptive Understanding technology, which enables the machine to become aware of its limitations and bail out to humans when it lacks confidence in its ability to provide the right answer, we at Interactions further improve the process.
Instances of Machine Learning Applications
Machine learning is widely employed across many industries and has a wide range of uses, particularly when it comes to gathering, analyzing, and responding to massive amounts of data.
The following list of significant uses for machine learning includes:
Healthcare
The use of machine learning is pervasive in this sector. Healthcare researchers can use it to assess data points and make recommendations. The accurate insights provided by natural language processing helped patients achieve better results. By evaluating outside data on patients’ situations, such as X-rays, Ultrasound, CT-scan, etc., machine learning has also enhanced therapy approaches. NLP, medical imaging, and genetic data are important applications of machine learning that advance healthcare’s diagnostic, detection, and prediction systems.
Automation
This is one of the key uses of machine learning that contributes to the system’s automation. Without human interaction, it enables machines to carry out monotonous activities. You have a responsibility as a machine learning engineer and data scientist to complete any given work repeatedly and without making any mistakes. For humans, this is not a realistic possibility. Since it can do iterative tasks faster, machine learning has created a variety of models to automate the process.
Banking and Finance
A branch of AI called machine learning makes precise predictions using statistical models. Machine learning was helpful in the banking and financial industry in a variety of ways, including fraud detection, portfolio management, risk management, and more. Consequently, machine learning is used extensively.
Prediction of traffic and transportation
Everyone uses this as part of their everyday routine, making it one of the most popular machine learning applications. It facilitates a variety of tasks, including highly secure routes, accurate ETA generation, vehicle breakdown prediction, and driving prescriptive analytics. Despite the fact that machine learning has resolved transportation issues, it still has to be improved.
Building an intelligent transportation system is made possible by statistical machine-learning methods. Deep Learning also investigated the intricate relationships between traffic, highways, roads, the environment, crashes, etc. In order to predict insights into routes and traffic, machine learning technology has enhanced daily traffic management as well as the collection of traffic data.
Image Recognition
One of the most popular uses of machine learning is image recognition, which is used to find images on the internet. Additionally, a tool on Facebook called “auto friend tagging recommendation” allows you to tag images with your Facebook friends using image recognition technology. Additionally, practically all modern mobile devices include fascinating facial-detecting features. In order to prevent unauthorized access to your mobile device, you can use this feature to safeguard your mobile data using face unlocking.
Speech Recognition
One of the greatest successes of machine learning applications is speech recognition. Users can “search by speech” or do text-free content searches using this technology. It may use your voice to search for information and items on platforms like YouTube, Google, Amazon, etc. Speech recognition is the name of this technique. Speech-to-text or computer speech recognition refers to the process of turning spoken instructions into written instructions. Google Assistant, Siri, Cortana, Alexa, and other voice recognition systems are some notable instances.
Stock Marketing and Trading
Machine learning is useful in the stock marketing and trading industry as well since it can predict market risk by using historical trends or prior knowledge. Share marketing is another word for marketing risk, which machine learning somewhat minimizes and foresees in data. Stock market movements are predicted using the lengthy short-term neural memory network of machine learning.
Our daily activities either directly or indirectly use machine learning. In the modern technological age, there are many machine-learning apps that are quite helpful for living. Despite still being in its infancy, machine learning is advancing quickly. The best feature of machine learning is its high-value forecasts, which, without human interference, may direct wiser decisions and proactive actions in real time. As a result, at the conclusion of this essay, we can state that the machine learning area is quite large and that its significance is not restricted to a particular industry or sector but rather is applicable everywhere for evaluating or forecasting future events.