Predictive Modeling for Banana Plantations: Enhancing Yield, Managing Diseases, and Timing Harvests
Banana cultivation is a vital component of the global agricultural landscape, providing a staple food for millions and supporting the livelihoods of countless farmers. In recent years, the agriculture sector has witnessed a technological revolution, with predictive modeling and analytics emerging as powerful tools for optimizing crop management. This article explores the application of predictive modeling in banana plantations, focusing on predicting crop yield, managing disease outbreaks, and determining optimal harvest times.
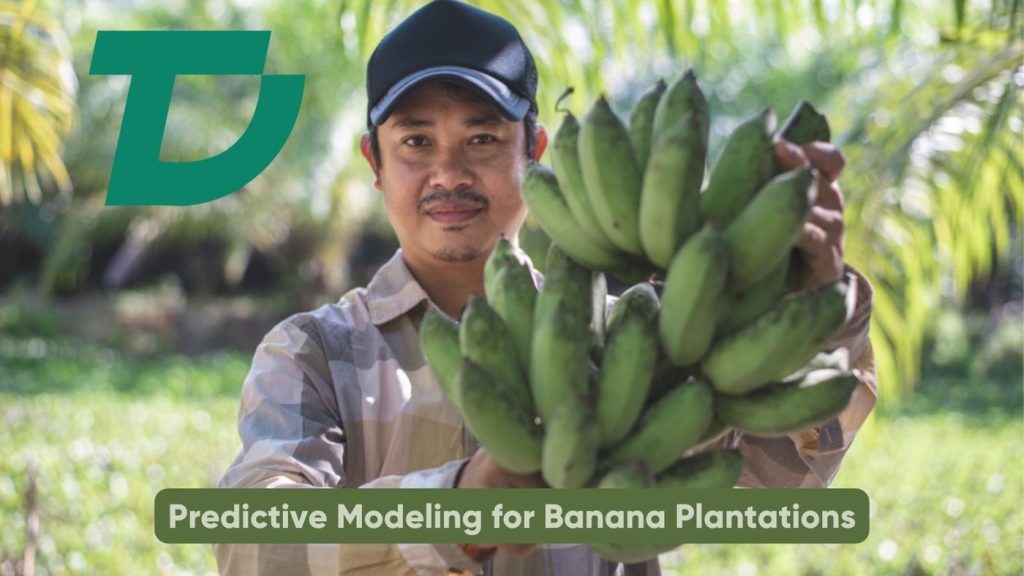
The Significance of Banana Crop Management
Banana plantations face numerous challenges, ranging from climate variability and soil conditions to the threat of diseases and pests. Efficient management is crucial to ensuring a bountiful harvest and maintaining the economic sustainability of banana farming. Predictive modeling plays a pivotal role in addressing these challenges by providing insights into various aspects of banana crop management.
Predicting Banana Crop Yield
One of the primary applications of predictive modeling in banana plantations is forecasting crop yield. By leveraging historical data, weather patterns, and soil conditions, predictive models can estimate the potential banana yield for a given season. This information is invaluable for farmers and stakeholders in making informed decisions regarding resource allocation, marketing strategies, and overall farm planning.
Data Sources for Predictive Yield Modeling
Predictive modeling relies on diverse datasets to generate accurate forecasts. These datasets include historical yield data, weather patterns, soil health indicators, and agricultural practices. Advanced sensors and satellite imagery contribute real-time data, enhancing the accuracy of predictive models.
Machine Learning Algorithms in Yield Prediction
Machine learning algorithms, such as decision trees, random forests, and neural networks, are employed to analyze the complex interplay of variables affecting banana crop yield. These algorithms learn from historical data patterns and use them to make predictions. As more data becomes available, these models continuously improve, offering increasingly accurate yield forecasts.
Benefits of Predictive Yield Modeling
- Resource Optimization: Farmers can optimize the use of resources like water, fertilizers, and pesticides based on predicted yields, reducing waste and minimizing environmental impact.
- Financial Planning: Accurate yield predictions enable farmers to plan their finances more effectively, securing loans or investments based on projected returns.
- Supply Chain Management: Stakeholders in the supply chain, including distributors and retailers, can make informed decisions about inventory and distribution, ensuring a steady supply of bananas to consumers.
Managing Disease Outbreaks
Banana crops are susceptible to various diseases, with Panama disease and Black Sigatoka being among the most notorious. Predictive modeling aids in disease management by identifying potential outbreaks, enabling timely interventions to mitigate the impact on crop yields.
Disease Data Integration
Predictive models incorporate data on disease prevalence, weather conditions conducive to disease development, and historical disease patterns. This information allows the model to identify high-risk periods and locations for disease outbreaks.
Early Detection and Prevention
Machine learning algorithms can analyze subtle patterns in the data that may indicate the early stages of a disease outbreak. Early detection allows farmers to implement preventive measures promptly, such as adjusting irrigation practices, applying targeted pesticides, or deploying resistant banana varieties.
Precision Agriculture for Disease Control
Predictive modeling supports precision agriculture, wherein interventions are tailored to specific areas of the plantation facing a higher risk of disease. This targeted approach minimizes the use of chemicals, reduces environmental impact, and ensures the most effective control measures.
Resilience Building
By continuously updating the predictive models with real-time data, farmers can enhance the resilience of their plantations. This proactive approach to disease management contributes to sustainable and robust banana farming practices.
Optimizing Harvest Times:
Determining the optimal harvest time is critical for maximizing banana quality, flavor, and shelf life. Predictive modeling assists farmers in making informed decisions regarding harvest timing, considering factors such as ripeness, weather conditions, and market demand.
Ripeness Prediction
Predictive models integrate data on banana maturation stages, historical harvest times, and environmental conditions to predict the optimal time for harvesting. This helps avoid premature or delayed harvesting, ensuring bananas reach consumers at peak ripeness.
Market Demand Forecasting
Understanding market demand is essential for banana farmers to plan their harvests effectively. Predictive modeling incorporates market trends, consumer preferences, and historical sales data to forecast demand, enabling farmers to align their harvest schedules with market needs.
Post-Harvest Handling
Predictive modeling extends its influence beyond the field by optimizing post-harvest handling processes. By predicting harvest quantities and ripeness levels, farmers can plan transportation, storage, and distribution more efficiently, reducing waste and enhancing the overall quality of bananas reaching consumers.
Challenges and Future Developments
While predictive modeling has shown great promise in revolutionizing banana plantation management, challenges persist. Data quality, accessibility, and the need for advanced technological infrastructure can pose barriers for some farmers. Additionally, continuous research and development are crucial to refining predictive models, incorporating new data sources, and adapting to evolving agricultural practices.
Data Challenges
Access to high-quality, real-time data remains a challenge for many banana farmers, especially those in remote or underdeveloped regions. Efforts to improve data collection infrastructure and promote data-sharing initiatives can help overcome this obstacle.
Technological Advancements
Continued advancements in sensor technologies, satellite imagery, and data analytics tools will enhance the capabilities of predictive models. Integration with the Internet of Things (IoT) devices and the use of drones for data collection are among the potential technological developments that could further revolutionize banana plantation management.
Climate Change Adaptation
As climate change poses new challenges for agriculture, predictive modeling must adapt to changing weather patterns and emerging threats. Collaborative efforts between researchers, farmers, and technology developers are essential to create resilient predictive models that can withstand the uncertainties associated with climate change.
Conclusion
Predictive modeling has emerged as a transformative force in banana plantation management, offering insights into crop yield predictions, disease management, and optimal harvest times. As technology continues to advance and agricultural practices evolve, the integration of predictive analytics in banana farming will become increasingly critical. By addressing challenges, embracing technological innovations, and fostering collaboration, the agricultural sector can harness the full potential of predictive modeling to ensure the sustainability and productivity of banana plantations worldwide.